Comprehensive, original, insightful, and otherwise interesting data science blogs
Most blogs in data science are like most people at a cocktail party: they talk news, weather, or politics, and do not venture into deep, interesting subjects. I provide a list of blogs that do.
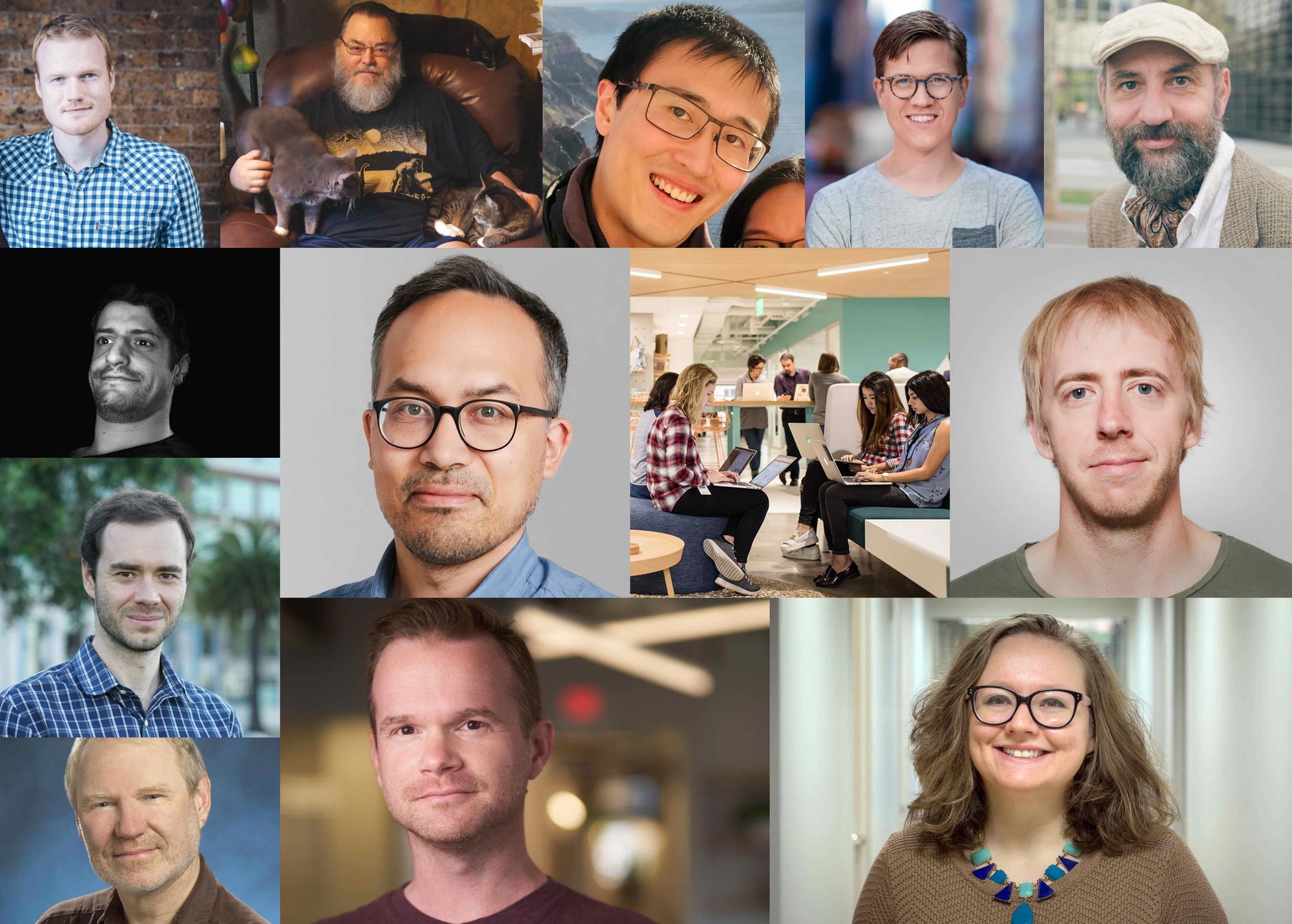
When I joined Narrator in March as a writer, I decided to spend some time researching what’s already written on data science/analytics to get a sense of the room that I had just entered.
The room turned out to be boiling with all sorts of blogs, Substacks, newsletters, and media publications. However, in most cases, the level of conversation there was similar to that of a cocktail party. (The party of SEO specialists who write for robots, not people.) Nevertheless, I still managed to find a few really interesting “people to talk to and learn from” and decided to share my list of these blogs publicly to spare others the trouble of digging the whole Internet quarry in search of a few nuggets of gold.
Before I announce my list, though, I want to outline my criteria for choosing these blogs and not others. What exactly do I mean by “comprehensive, original, insightful, and otherwise interesting?”
- Comprehensive. Instead of writing “3 tips” or “5 steps” fluff, comprehensive authors treat their subject as deeply and wholly as possible, always prioritizing the evergreen, abstract, and fundamental over journalistic, concrete, and shallow.
- Original. Almost self-explanatory: original authors share their own thinking instead of refurbishing someone else’s ideas. (This might range from something as simple as offering one’s opinion on a certain matter, as Tristan did here, to writing a totally new theoretical work, as Andrej did here.)
- Insightful. Insightful authors don’t just report the facts; they explain why these facts are the case and how they connect to other things. By reading their (often very dense) writing, you have many “huhs” per paragraph. They show you how one piece of the puzzle connects to everything else and help to form a better, more coherent model of the world. (Incidentally, the number of “huhs” per paragraph is a good measure for both objective quality and subjective value of writing.)
- Otherwise interesting. Most of the authors on the list, being genuinely curious, have remarkably broad interests. As a result, they don’t write just about data; they write about all sorts of things and often, as Vicki put it, “…view tech from the lens of how it works for different companies, and through data…” and “…like thinking about larger trends and noodling over what they mean for us all.” That’s what makes them stand out.
In addition to these four, I tried to select blogs that have been writing consistently since they started, be it two decades or just five months ago. (You wouldn’t want that amazing person you just met at the cocktail party to leave unannounced five minutes later, would you?)
Stylistically, I opted for blogs with clear, concise, and long-form writing. There are two reasons for that. First, clear and concise writing is similar to a large and clean window on a train; it offers you a complete, undistorted picture of what’s out there and does not distract you with blobs and dirt. Second, long-form, distraction-free nonfiction is a remarkable tool for thought because it promotes understanding through sustained concentration.
With that, here’s my unordered list of comprehensive, original, insightful, and otherwise interesting data science blogs:
- Benn Stancil, benn.substack and Mode Blog
- Tristan Handy, dbt blog, Medium, and RJMetrics blog, as well as some posts from The Analytics Engineering Roundup
- Petr Janda, petr@substack
- Mikkel Dengsøe, Inside Data by Mikkel Dengsøe
- Mikio L. Braun, Marginally Interesting one and two
- StitchFix team, MultiThreaded
- Vicki Boykis, Tech Blog and Normcore Tech
- Randy Au, Counting Stuff
- Erik Bernhardsson, blog
- Charlie Kufs, Stats With Cats Blog
- Stephen Few, Visual Business Intelligence
- Martin Fowler, martinFowler.com
- Andrej Karpathy, Andrej Karpathy blog and Medium
In the rest of the post, I will go through each of them in detail, explain why I think they’re a good fit for this list, offer some quotes to help you get a sense of each author, provide a list of my favorite posts, and supply you with many valuable links. Finally, I’ll share some peculiar observations I made during the past month of blog research. I hope you’ll find them useful.
P.s. If you know of any other comprehensive, original, insightful, and otherwise interesting data science blogs, please email me at vasili at narrator.ai, and I’ll gladly consider adding them to the list. My goal is not to own this list but to make it useful.
Benn Stancil
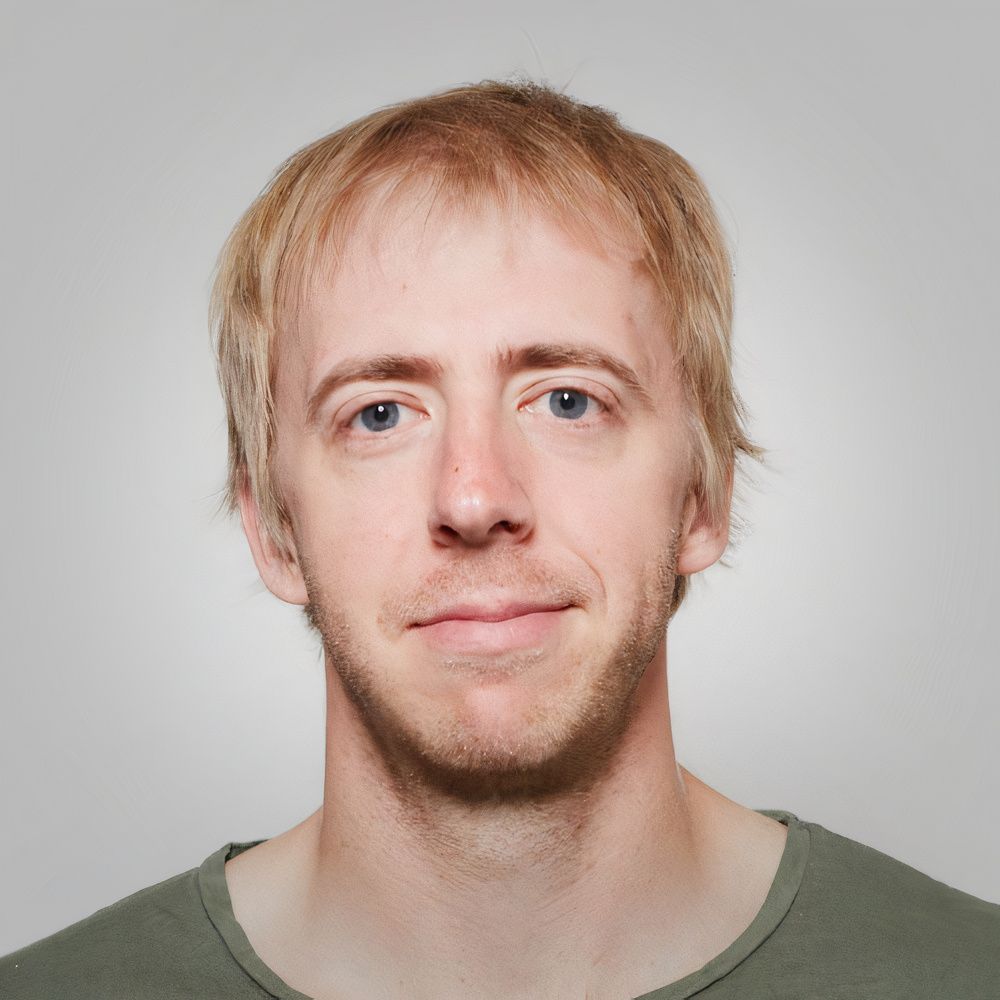
There’s no better candidate for opening the list than Benn Stancil, for his Substack manifests all four criteria. Armed with decades of experience and crisp literary style, Benn writes profound, almost philosophical works about data, analysis, data industry, and much more.
Here are some appetizers to get a taste of Benn’s writing:
- The stock market is down again, and that can only mean two things: First, woo woo technical analysts are posting candlestick charts of stock prices, randomly drawing lines between points, circling the days when Mercury was in retrograde, calling it “the death cross,” and announcing that it’s time to buy again because the 13-session countdown pattern has broken a resistance band; and second, venture capitalists are falling over themselves to tweet out apocalyptic R.I.P Good Times predictions. (full post)
- To most people—pleasant, social people, the kind who can make it through a party without arguing about SQL formatting—the modern data stack isn’t an architecture diagram or a gratuitous think piece on Substack or a fight on Twitter. It’s an experience—and often, it’s not a great one. It’s trying to figure out why growth is slowing before tomorrow’s board meeting; it’s getting everyone to agree to the quarterly revenue numbers when different tools and dashboards say different things; it’s sharing product usage data with a customer and them telling you their active user list somehow includes people who left the company six months ago; it’s an angry Slack message from the CEO saying their daily progress report is broken again. (full post)
- Decision makers, from CEOs to the person on the couch with the remote, also use data to serve a more subtle purpose: As a substitute for courage. In these cases, we don’t use data to lie to others; we use it to lie to ourselves. (full post)
In addition to his Substack, Benn writes on his company’s blog. Check it out if you want to read more of his works. (Unfortunately, there’s no way to filter posts by author there, so you’ll have to either Cmd+f “benn” or Google “benn mode blog”.)
Here’s my favorite posts from Benn:
The last one has a comment on Substack to which I fully subscribe:

Tristan Handy
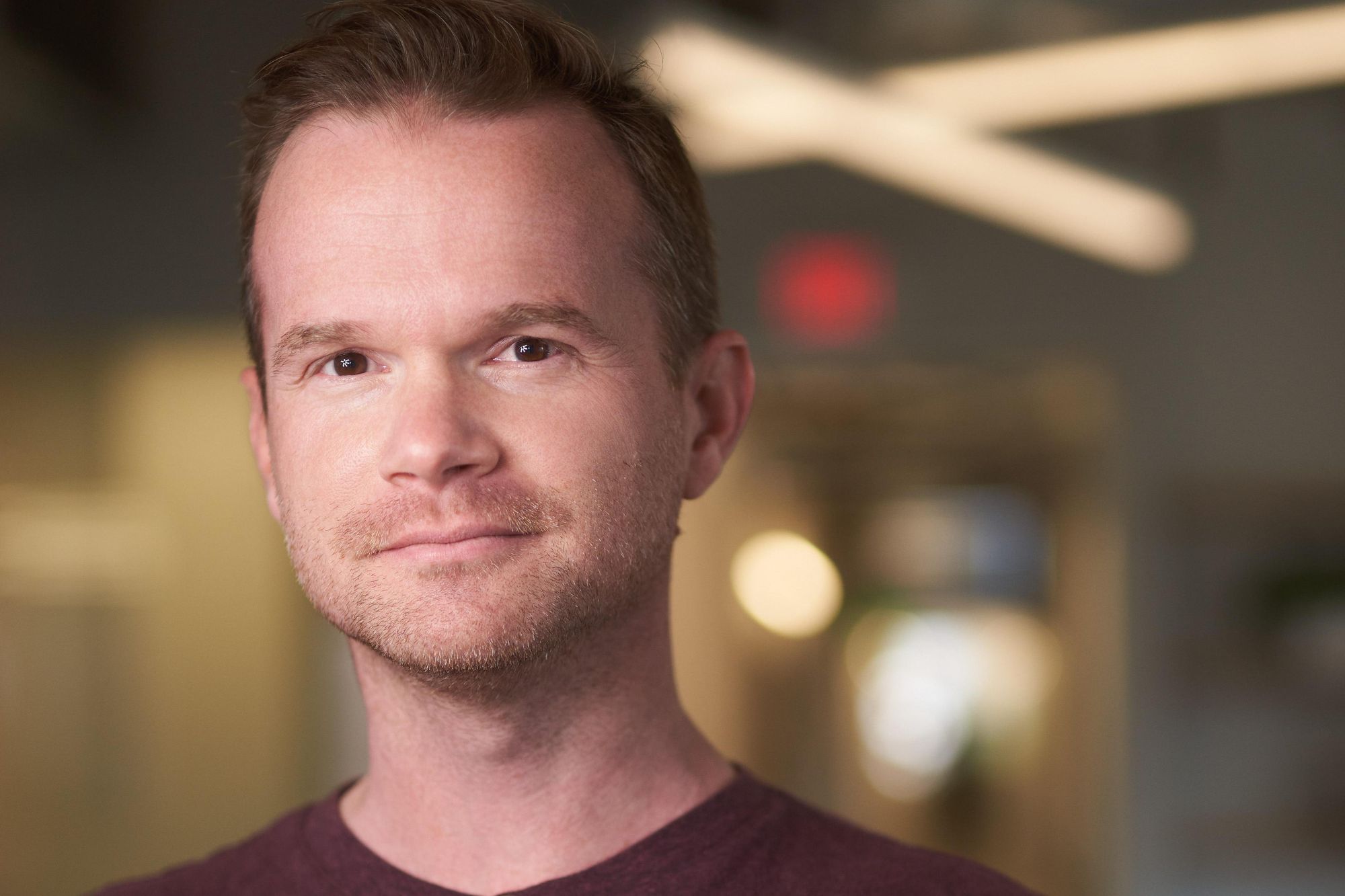
The second author I found interesting is Tristan Handy, the founder of dbt Labs who writes primarily on dbt Blog and The Analytics Engineering Roundup newsletter. He is a prolific writer and has been sharing his thoughts publicly long before he started dbt; first on the blog of RJMetrics, another company of his, and then on his personal Medium blog. You may check out them all.
Some bites of Tristan’s work:
- If you fell asleep, Rip Van Winkle-style, in 2016 and woke up today, you wouldn’t really need to update your mental model of how the modern data stack works all that much. More integrations, better window function support, more configuration options, better reliability… All of these are very good things, but they suggest a certain maturity, a certain stasis. What happened to the massive innovation we saw from 2012-2016? (full post)
- Growing a company is like a poker tournament: you might win a couple of lucky hands early, but ultimately you’re going to have to make good decisions if you want to win the whole thing. And good decisions are founded on a solid understanding of facts. (full post)
- Now that this technology is being built modularly, targeting widespread adoption and with a focus on open source and open standards, I believe we will see steady forward progress towards recognizing the longstanding dream of listening, processing, and reacting to company data using a single cohesive set of technologies. I know that sounds lofty. I tend to like to keep my prognostications rather more grounded, but I really do believe this is the direction the industry is moving. If you disagree, please, call bullshit in the comments. (full post)
Tristan writers in a friendly, casual, and somewhat emotional style. However, this style does not make him a shallow writer. He treats the subject comprehensively and produces fascinating industry overviews and well-thought opinion pieces that manage to yield those “huhs” every few paragraphs.
Here’s my favorite posts from Tristan:
- The Modern Data Stack: Past, Present, and Future
- Scaling knowledge
- The Data Infrastructure Meta-Analysis: How Top Engineering Organizations Built Their Big Data Stacks
- Macroeconomics and the data industry
You may also like reading his four year-in-review posts about their progress with dbt Labs. I’ve found them helpful to form a better understanding of both dbt and the modern data industry:
- One year in: we're still in business (Jun 30, 2017)
- Two years in and it's just getting good (Jul 5, 2018)
- Three years in: things are heating up (Jul 2, 2019)
- Four Years In: From Misfits to Mainstream (Jul 2, 2020)
Petr Janda
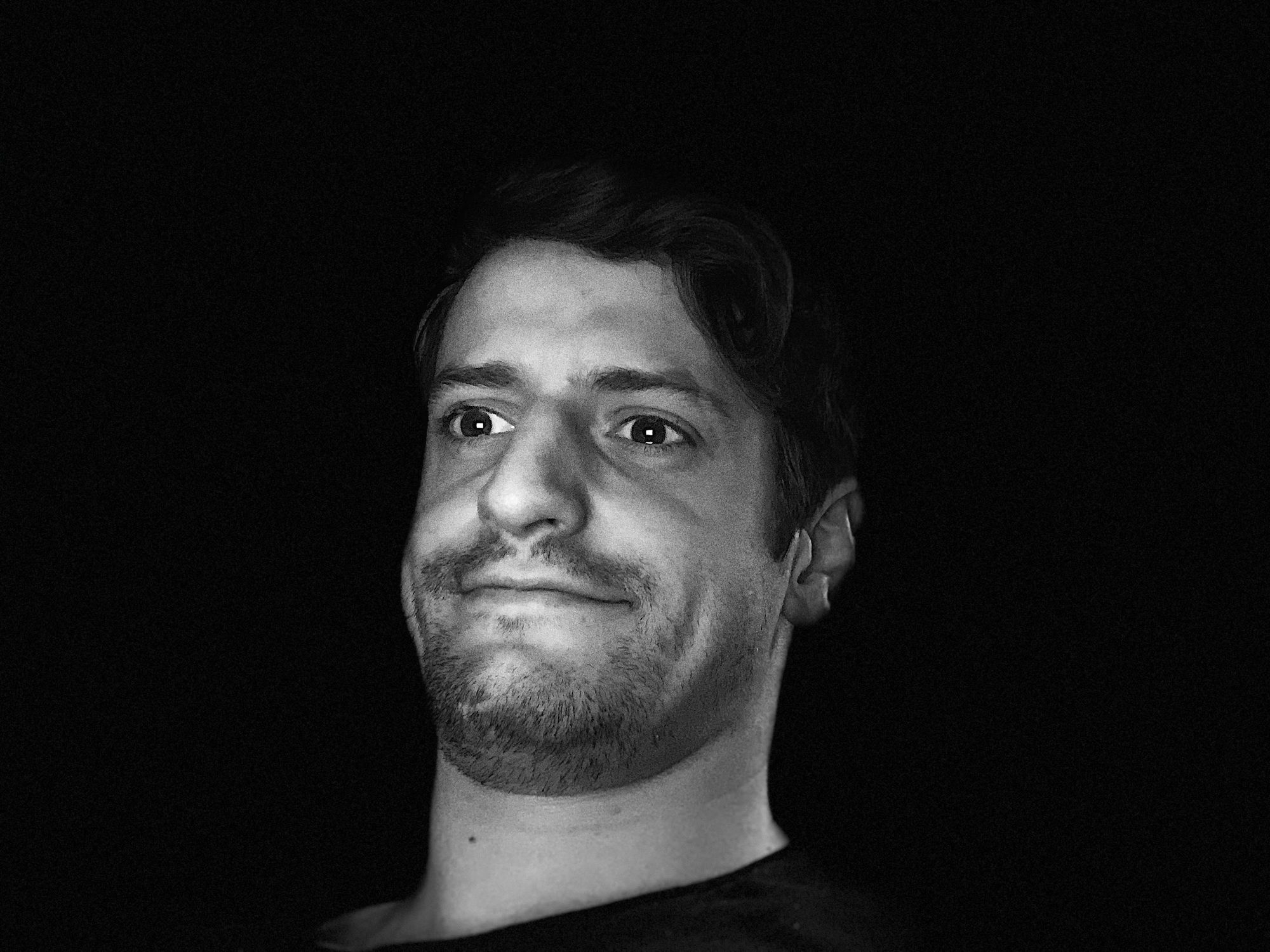
In comparison to Benn and Tristan, who have been writing for decades, Petr, who published his first Substack post, The messy core of the Modern Data Stack, on Oct 8, 2021, is just getting started. However, this does not make his writing even a gram less interesting than the two. He writes comprehensively, clearly, tells good stories, provides tons of facts, and primarily covers the role of a data analyst in a modern data team and the modern data stack itself. You can check out his Substack here.
To get a taste of his writing, sample these bites:
- In today’s business, on average, 110 SaaS tools produce data every single day. Before the Modern Data Stack emerged, this data was siloed. A typical company didn’t have an army of engineers to build custom pipelines for every single tool. Data sitting in these tools was out of reach. Not any more. The tools like Fivetran3, Airbyte4 plug the majority of this data into the data warehouse. And with access, the expectations of the business grew too. (full post)
- The Modern Data Stack initially feels simple, so we run a few scripts, do a few clicks here and there to set it up. But it becomes increasingly hard to maintain as the company scales. It turns out it becomes very similar to other software: more code, configuration, components, and scripts. And since we have a much longer history managing our Software, we should explore if we can learn from it. (full post)
- In 1975, 83% of the value of SAP500 companies lay in their tangible assets. The remaining, 17%, were intangible. This is no longer the case. In 2015 the ratio reversed, and in 2020 average percentage of tangible assets dropped as low as 10%. Today, software companies powered by data dominate the bench of corporate giants. (full post)
Here’s my favorite posts from Petr:
- Why the Data Analyst role has never been harder
- Why should we “refuse to click buttons”
- How to get more power from your data analytics engine
Mikkel Dengsøe
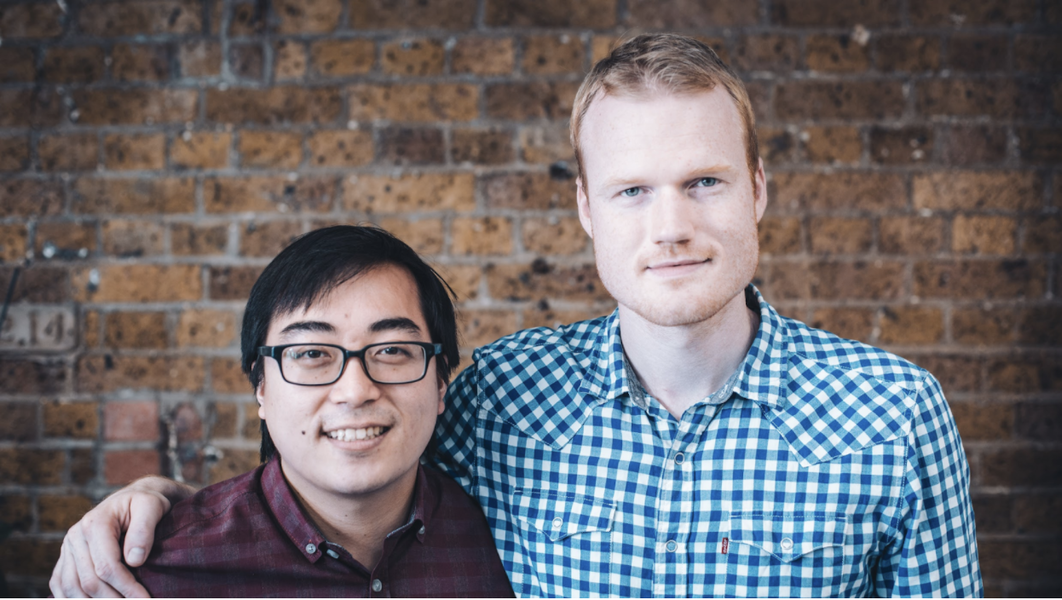
Mikkel Dengsøe, who started publishing his work at the same time as Petr, also writes about data analysis and the industry more broadly. However, unlike Benn, Tristan, and Petr, he has a different weapon: masterful analogies, metaphors, and visuals.
For example, consider his post Moldy data and dashboards: safe to eat? He suggests that dashboards are kind of like bread: they grow moldy over time. In an instant, you get a good idea of what he’s talking about, especially if you see the analogy visually:
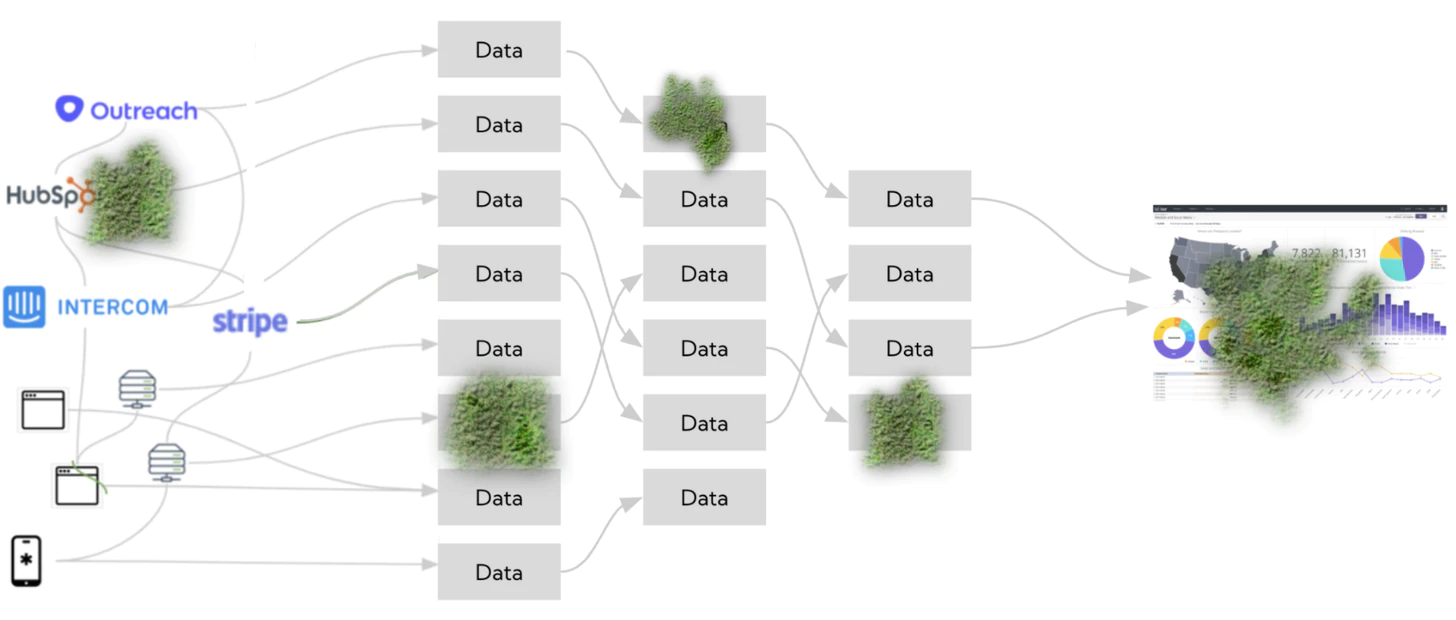
Some appetizers for Mikkel’s work:
- Moldy data deteriorates trust in data and leads to a situation where more time in meetings is spent discussing what data means and if it’s right rather than making informed decisions based on it. (full post)
- Spreadsheets are fragile: If dashboards feel like a house made of solid bricks, spreadsheets are made of thin sticks held together by duct tape. Something temporary that’s never 100% accurate and more often than not have a few calculation errors. (full post)
- If you peek under the hood of a modern company's data stack you’ll most likely find all the usual suspects; dbt, Airflow, Fivetran, BigQuery and Looker. But one guest always shows up to the party uninvited. Hello Mr. Spreadsheet. (full post)
He also likes writing comprehensive industry overviews, which I’ve found to be my favorite posts of his:
- We’ve only scratched the surface of the full potential for the data warehouse
- Data to engineers ratio: A deep dive into 50 top European tech companies
- Data salaries at FAANG companies in 2022
Mikio L. Braun
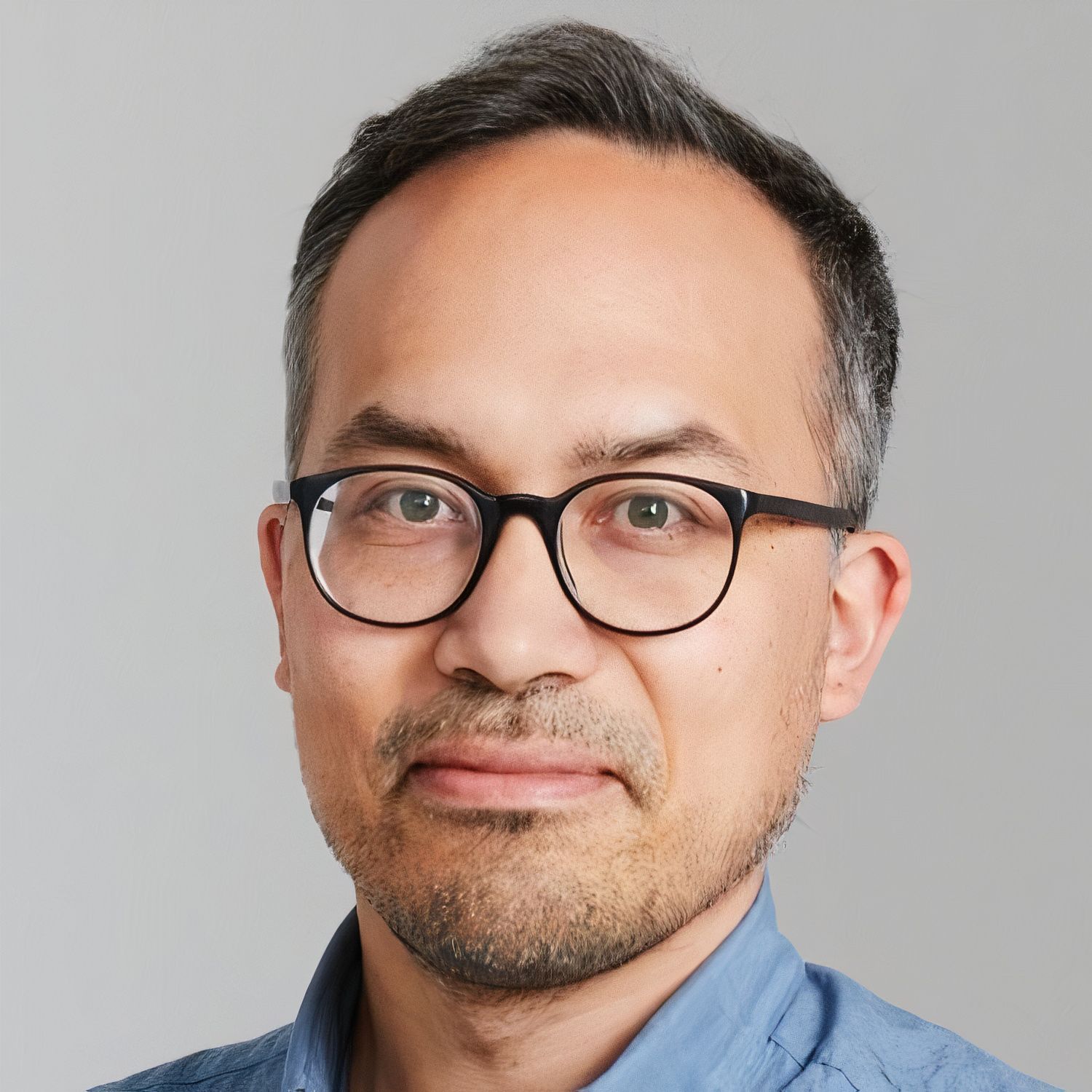
Mikio is a machine learning consultant who has been consistently writing about ML, data science, and data analysis for the past 16 years. His writing is much more technical than that of the authors above, but still accessible (and super interesting) to the general reader. He likes to drill down to the very essence of things and explain that precious tacit knowledge that often eludes us. You can read his blog here.
For example, consider his post Three Things About Data Science You Won’t Find In the Books from 2015. Don’t get fooled by the seemingly shallow title. Give it a read. Likely, you’ll feel like one of the commentators:

Here’s some more appetizers to Mikio’s work:
- I don’t know whether this word exists, but mainstreamification is what’s happening to data analysis right now. (full post)
- The general message is: Data analysis has become super easy. But has it? I think people want it to be, because they have understood what data analysis can do for them, but there is a real shortage in people who are good at it. (full post)
- Tools only provide you with possibilities, but you need to know how to use them. (full post)
Mikio also has an index of all posts from his old blog, which you can skim through in 1-2 minutes to identify the most relevant posts for you from his whole body of work from 2005 to 2017. (Blogs should have more of these things!)
Here’s my favorite posts from Mikio:
- Data Analysis: The Hard Parts
- AI's Road to the Mainstream (A totally subjective history of the past 20 years)
P.s. He just published a new post on Apr 8!
StitchFix team

StitchFix’s MultiThreaded blog is the most interesting company blog that I’ve found. Unlike many other company blogs, which are full of shallow “7 tips” and generic “how-to” fluff, this one abounds with unique, original works from the team’s personal experience at StitchFix. Furthermore, these posts are written in a personal, clever, warm, and friendly style, and masterfully connect theory with practice.
Here’s a sneak peek:
- While the proliferation in the amount of data modern businesses collect has created many opportunities, it has also introduced challenges for sound decision-making. In some organizations, earnest efforts to be “data-driven” devolve into a culture of false certainty in which the influence of metrics is dictated not by their reliability but rather by their abundance and the confidence with which they’re wielded. (full post)
- Looking at more data on more things doesn’t necessarily produce better decisions; in many cases, it actually leads to worse ones. (full post)
- Perfect execution on requirements and complacency brought on by achieving process efficiencies can mask the difficult truth, that the organization is blissfully unaware of the valuable learnings they are missing out on. (full post)
My favorite posts:
- The Sobering Truth about the Impact of your Business Ideas
- Beware the data science pin factory: The power of the full-stack data science generalist and the perils of division of labor through function
Vicki Boykis
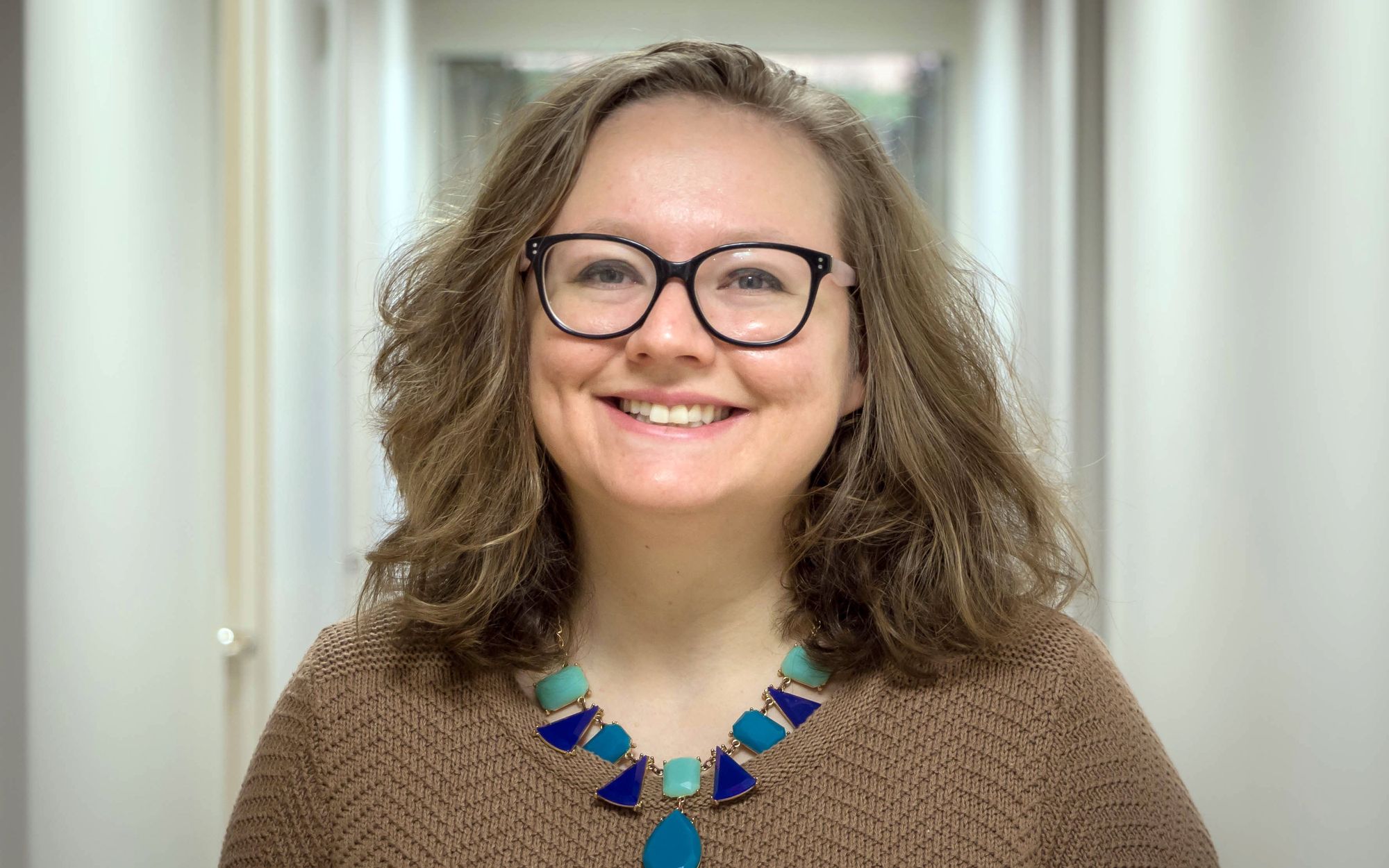
Soon after I began writing Vicki’s profile for this post, I realized that it’s a fool’s errand. It is hard to describe Vicki better than she did, so I will just paste her own “about me” here:
“I’m Vicki, and I like to think about technology in all sorts of different ways. At work, I’m a data science consultant working with a wide variety of different companies, so I view tech from the lens of how it works for different companies, and through data. I like thinking about larger trends and noodling over what they mean for us all.”
If my criteria for this post had 0-10 scores, Vicki would score 11/10 for “insightful.” Her blogs are incredibly intertwined with each other and with writings of other people, which makes reading her work is an endless stream of “hahs” and “huhs.” You can read her Tech Blog here.
Here’s a sneak peek:
- What makes it harder is that dealing with data is like dealing with a moving river (perhaps that’s the reason we’ve developed so many water terms around our data processes - data lakes, streams, and putting all of it in the cloud.) Data is ephemeral and constantly shifting as users input data, engineers log different things, and you gain or lose access to storage. (full post)
- The final thing I’ve learned is that companies operate mostly on gut instinct. Many will tell you that they’re data-driven. People love this idea, of making decisions based on The Numbers, and want to believe it about themselves. I want to believe this about myself! But oftentimes, data will serve mostly as supporting evidence for a gut check, and the gut check depends on a lot of factors, including whether you trust the person presenting the data, what your view of the company is, and what corporate politics are at play. (full post)
- So what I do I do every day? I like to think of myself as Benedict Cumberbatch…the lone genius working on very complicated puzzles. But in reality, a lot of a data scientist’s daily work involves making sense of and cleaning data in order to get it ready for analysis. So my day-to-day involves a little of each of the three areas: programming, stats analysis, and domain expertise; I.e. knowing the business and the data associated with it. No one data scientist is good at all three; most will be very good at two. That’s why it’s good to have a team with complementary skills. (full post)
In addition to her blog, Vicki also writes a popular Substack called Normcore Tech. In it, she goes beyond data and covers “how systems work, online advertising, Moana, and the deceptiveness of our public personas.” Check it out.
You can find indexes of all her blog posts for the past 7 years here (for main blog) and here (for Normcore Tech Substack).
My favorite posts from Vicki:
Randy Au

Randy has a weird job title: Quantitative UX Researcher. Probably that’s why the only common denominator behind his extensive writings is just that: quantitative work. What makes him relevant to my list, though, is his mastery of integration. In his own words: “There’ll also be frequent forays into technical topics, especially those that are important to understand when … counting stuff. But expect the occasional poke at topics that are tech-adjacent and just plain interesting and nerdy.” That’s the kind of writing you want to read if your aim is understanding.
Like Mikkel, Randy is a master of analogy. His writing is clear, concise, and distraction-free, which is so important in the today’s noisy world.
Some appetizers from Views on dashboards being answers, or not:
- Since dashboards are like fixed panes of glass that provide a particular view out into the world, they’re generally consistent over time in what they show. This property of providing a single consistent view of the world in theory allows observers to notice that “hey, things aren’t heading in the right direction, we need to make a course correction.” This is very much like the windshield of your car, it consistently shows what is in front of you and if you notice that you’re starting to veer into a tree, you make adjustments.
- The topic of what “self-serve” means in a data/information context is a bit polarizing. We can all think of horrible examples from both ends of the spectrum, with people who could’ve used data access to avert disaster not having it available, as well as people who abused access to data to come to very bad conclusions.
- [Dashboards] are never at the right ‘altitude’. They’re either too high level, or too detailed.
My favorite posts:
Erik Bernhardsson

Once in a while, I stumble upon a blog post so good that I immediately lose sight of what I was supposed to be doing and begin hurriedly scrolling the other posts by the same author because I have a hunch that I just entered one of those rare goldmines of knowledge on the Internet. Erik’s blog is such a goldmine.
In his own words, “Erik Bernhardsson is the founder of Modal Labs which is working on some ideas in the data/infrastructure space. I used to be the CTO at Better. A long time ago, I built the music recommendation system at Spotify. You can follow me on Twitter or see some more facts about me.”
He is one of those rare people who write broadly, deeply, and consistently. (He has been publishing for 10 years already.) Almost every blog post of his is an example of what it means to treat the subject well. His style is similar to that of Tristan; both write personally, cleverly, and simply.
Here’s some appetizers:
- I’m all for specialization! The society has come a long way from subsistence agriculture and that’s almost objectively a good thing. The economy organizes people into different trades and different professions and let’s people benefit from their comparative advantage. (full post)
- Big transformations tend to happen in two stages. The first step happens when some new technology arrives and people adopt to it in the simplest way that lets them retain their conceptual models from the existing world. The real transformations happens later, when you rethink the consumption model because the new world opens up new ways to create value. The way we consumed music didn't change materially when Apple started selling songs online. The real transformation happened when providers like Spotify realized the whole notion of ownership didn't matter anymore. (full post)
- Ideas will be generated much faster than there's bandwidth to execute on them, so you're doing something right if your backlog is growing indefinitely. A negative person on a mediocre team will complain that there's never time to work on their favorite pet project X. I've often heard things like “our backlog of features keeps growing so fast, how are we ever going to have time to invest in paying down tech debt?". To me this reflects a misunderstanding of how product development should work. Backlogs should be growing indefinitely. What a good team will do is to accept that, and establish a good relationship between product and tech, and make sure you constantly keep reprioritizing. Maybe today it's shipping a bunch of features the business needs. Maybe tomorrow it's paying down some tech debt. If you have a shared framework for how to think about value and prioritization, it usually works out. (full post)
My favorite posts:
- What is the right level of specialization? For data teams and anyone else.
- Building a data team at a mid-stage startup: a short story
- Storm in the stratosphere: how the cloud will be reshuffled
You can review all his top posts since 2014 here.
Charlie Kufs

Even though Charlie does not write about the data industry per se, I think his Stats With Cats Blog is still a gem for anyone working in data for a simple reason: he writes about statistics, which is a crucial part of data science (and, more broadly, making sense of the world).
He writes comprehensively yet personally, connects things that you’d not even think of connecting, provides extensive tables of contents for big posts (I’d love more of those in other blogs!), and, as you may have already guessed from the title, does all that with cats. What a treat!
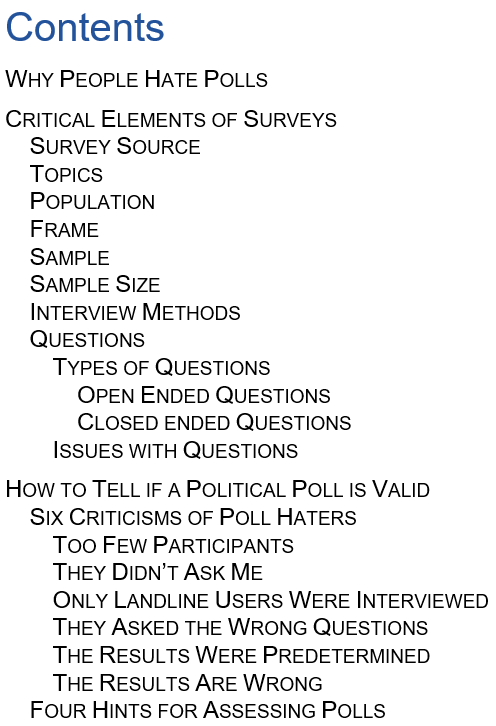
Here’s a sneak peek to his work:
- History isn’t always clear-cut. It’s written by anyone with the will to write it down and the forum to distribute it. (full post)
- Every organization wants to believe that they use information to make decisions in an unbiased manner, although not every organization actually does that. (full post)
My favorite posts from Charlie:
Stephen Few
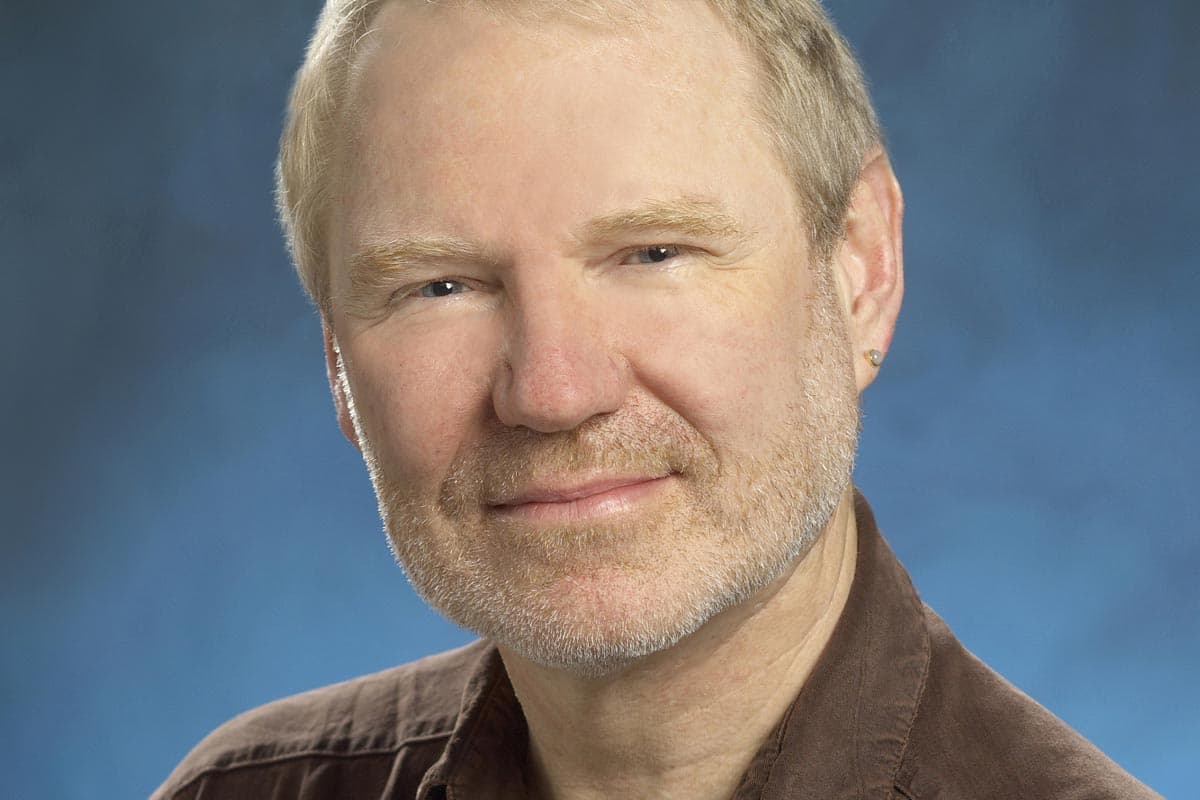
Perhaps the best way to introduce Stephen’s blog is through a screenshot from his main site:
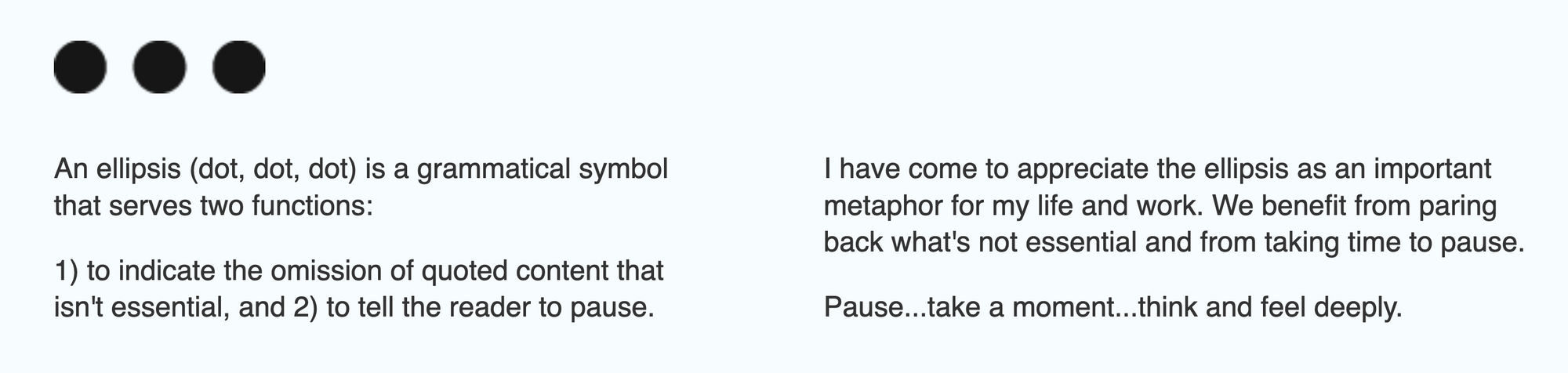
This is exactly how reading Stephen’s posts feels like. Pause. Think. Feel deeply.
Having worked for 25 years in data visualization, he writes about “the practical uses of data visualization to explore, analyze, and present quantitative business information.” Stephen is similar to Charlie: both do not write about “data” or “data science” directly but cover a crucial, fundamental aspect of it, be it statistics or visualization. However, being a genuinely curious person, he also ventures into topics like art, big data, and principles of design. He even writes book reviews! (71 so far.)
Appetizers:
- No analytical technologies or technical skills will overcome a scarcity of sound reason. (full post)
- For years a battle has raged between infographic designers who emphasize the importance of aesthetics and data visualizers with a more practical bent who focus on the degree and quality of understanding that results. Those in the aesthetics camp argue that if an infographic is not eye-catching, no one will look at it, and that compromises in the quality of communication are justified as a means to capture the reader’s attention. Those in the optimal-understanding camp argue that the reader’s attention is wasted if the visualization does not clearly and accurately tell its story. In truth, most people have joined one camp of the other, not because of deep thinking on the topic, but because of preferences formed by their experience or lack of it. (full post)
- Because I’ve been thinking a lot about so-called Big Data these days, I couldn’t help but see a connection between Big Data and this pile of peanuts. Just as this pile of nuts is an incremental representation of the artist’s days on earth, Big Data is an incremental increase in data volume, velocity, and variety. Two differences exist, however. The Big Data pile of nuts increases at an exponential rate, unlike the linear increase of the artists lifespan, and the artist hasn’t chosen to give herself a new name for arbitrary points along the growth of this pile. Data has been increasing at an exponential rate since the advent of the computer; it didn’t suddenly become big and it hasn’t passed some threshold that makes is qualitatively different than it was in the past. (full post)
My favorite posts from Stephen:
- Data Analysts Must Be Critical Thinkers
- Should Data Visualizations Be Beautiful?
- Big Data: A Pile of Nuts
You can view a topical index of all his writings for the past 17 years here, and the rest of his content (books, articles, whitepapers, and other brief publications) here, in his library. I also recommend inspecting his latest book, Now You See It, Second Edition. It is the most comprehensive read on the subject of data visualization or, as Stephen calls it, “visual data sensemaking,” that I’ve seen.
P.s. Unfortunately, Stephen stopped publishing new posts in Feb 2021. I hope he is doing well.
Martin Fowler
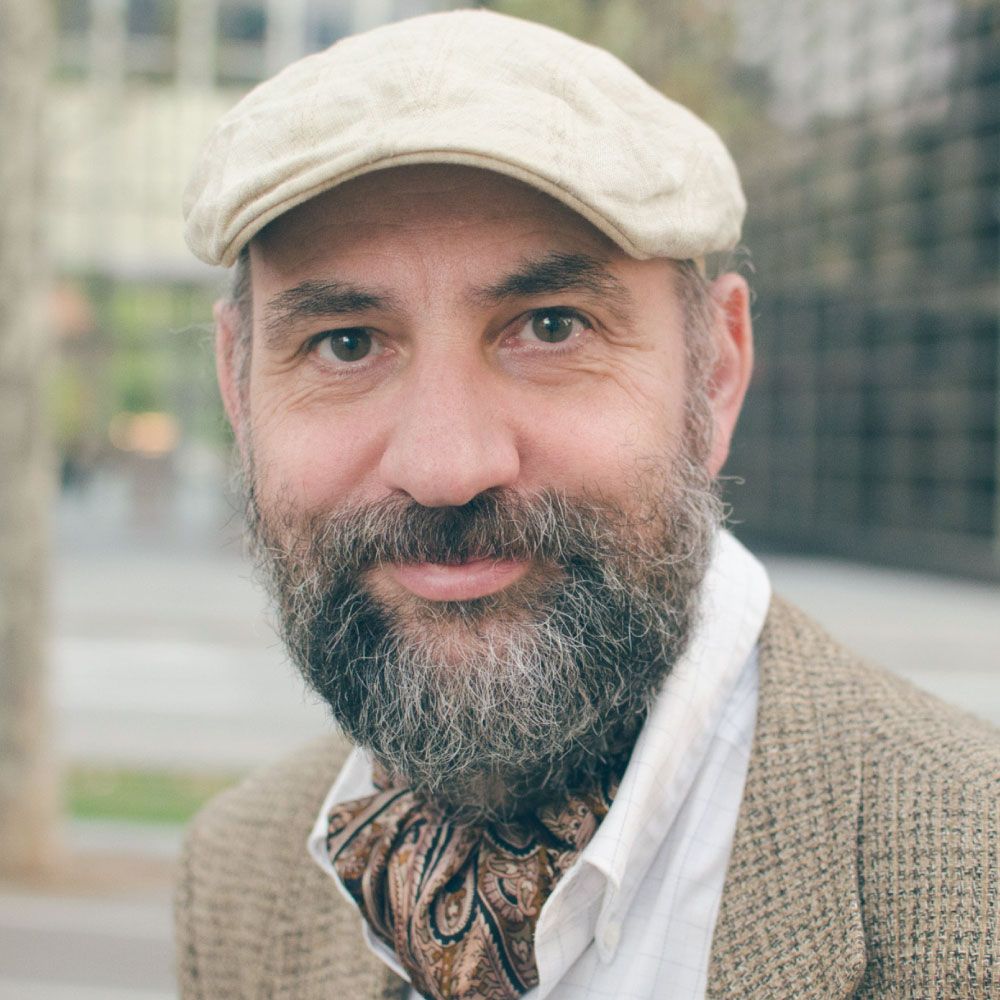
Martin Fowler is a legend in the software world who needs no introduction. However, few people know that amongst the stupendous 817 items of content he has published in the past X years on his site, a great deal is about data and data science. He even has a dedicated Data Management Guide and an interactive presentation on Big Data, which I highly recommend to anyone who’s just dipping their toes into the field. You can read his blog here.
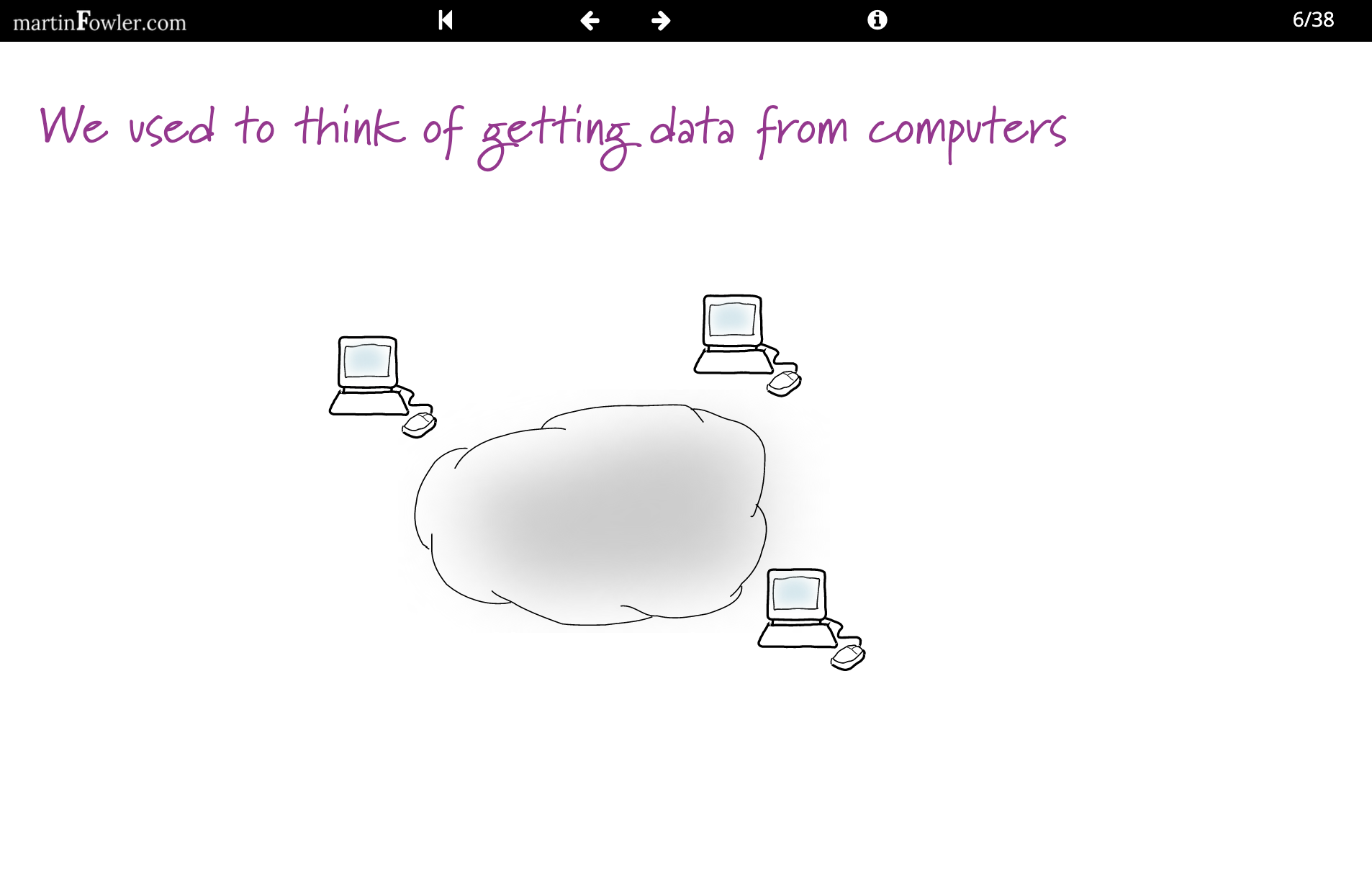
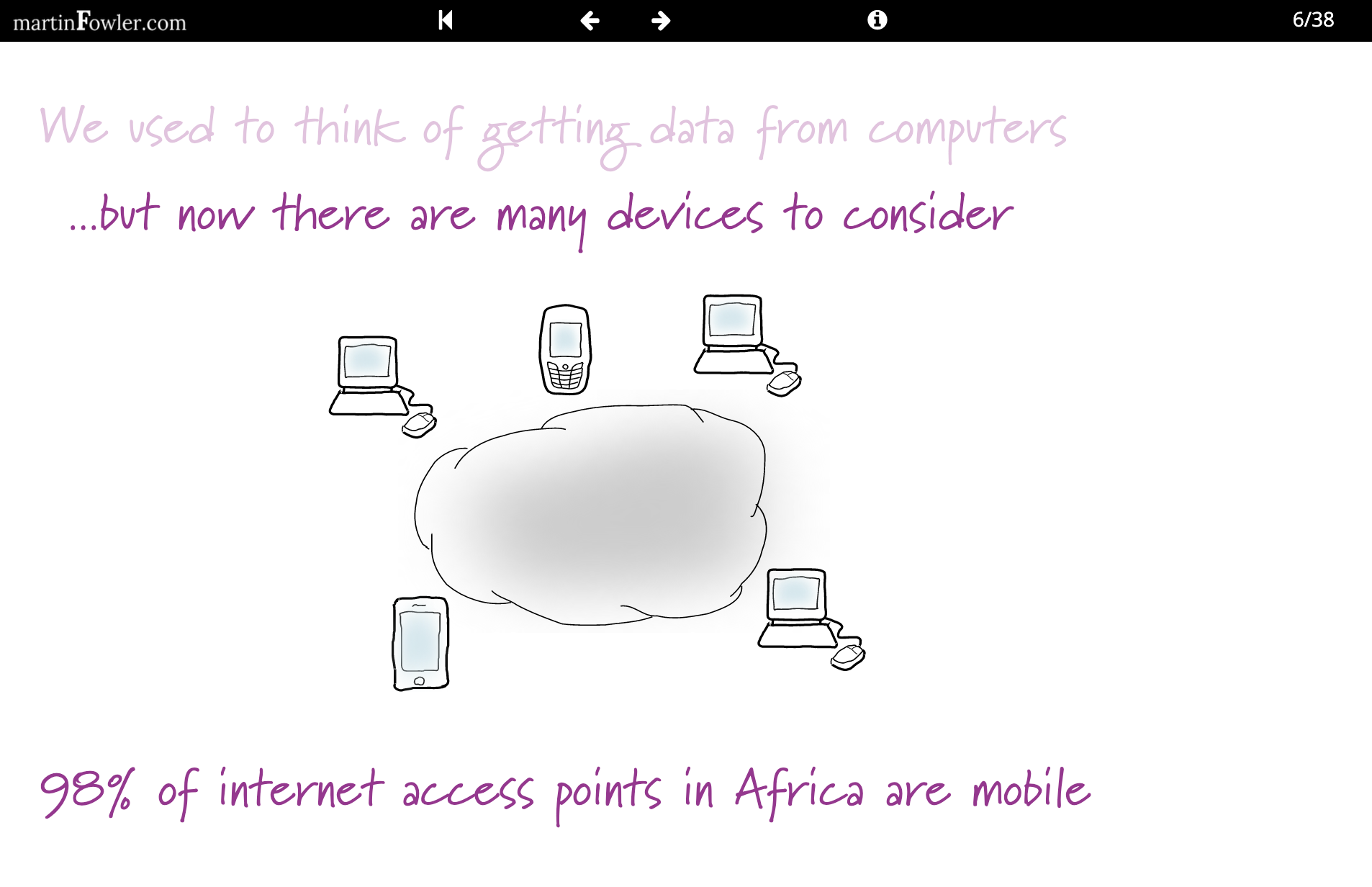
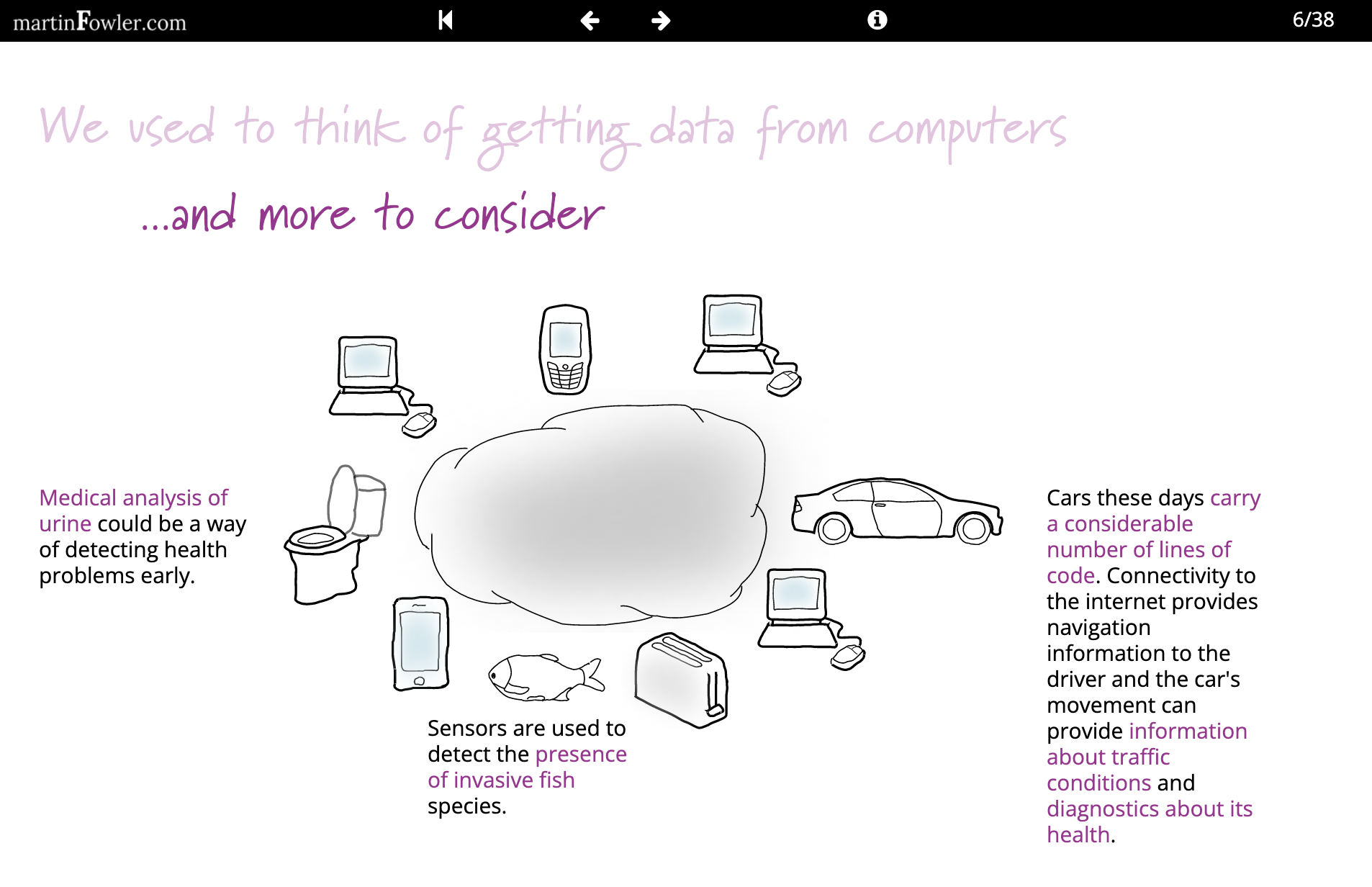
(Incidentally, I’d argue that reading comprehensive, in-depth writings on software and agile from Martin might be more valuable for a data person than reading shallow, fluffy posts about data science from some random magazine.)
Here’s some delicious bites from Martin’s blog:
- It’s natural to wonder what will happen over the next few years, will computers soon have greater intelligence than humanity? (Given some recent election results, that may not be too hard a bar to cross.) (full post)
- But as I hear of these, I recall Pablo Picasso’s comment about computers many decades ago: “Computers are useless. They can only give you answers”. The kind of reasoning that techniques such as Machine Learning can result in are truly impressive in their results, and will be useful to us as users and developers of software. But answers, while useful, aren’t always the whole picture. I learned this in my early days of school - just providing the answer to a math problem would only get me a couple of marks, to get the full score I had to show how I got it. The reasoning that got to the answer was more valuable than the result itself. That’s one of the limitations of the self-taught Go AIs. While they can win, they cannot explain their strategies. (full post)
My favorite posts:
You can review an alphabetical index of all Martin’s content here.
Andrej Karpathy
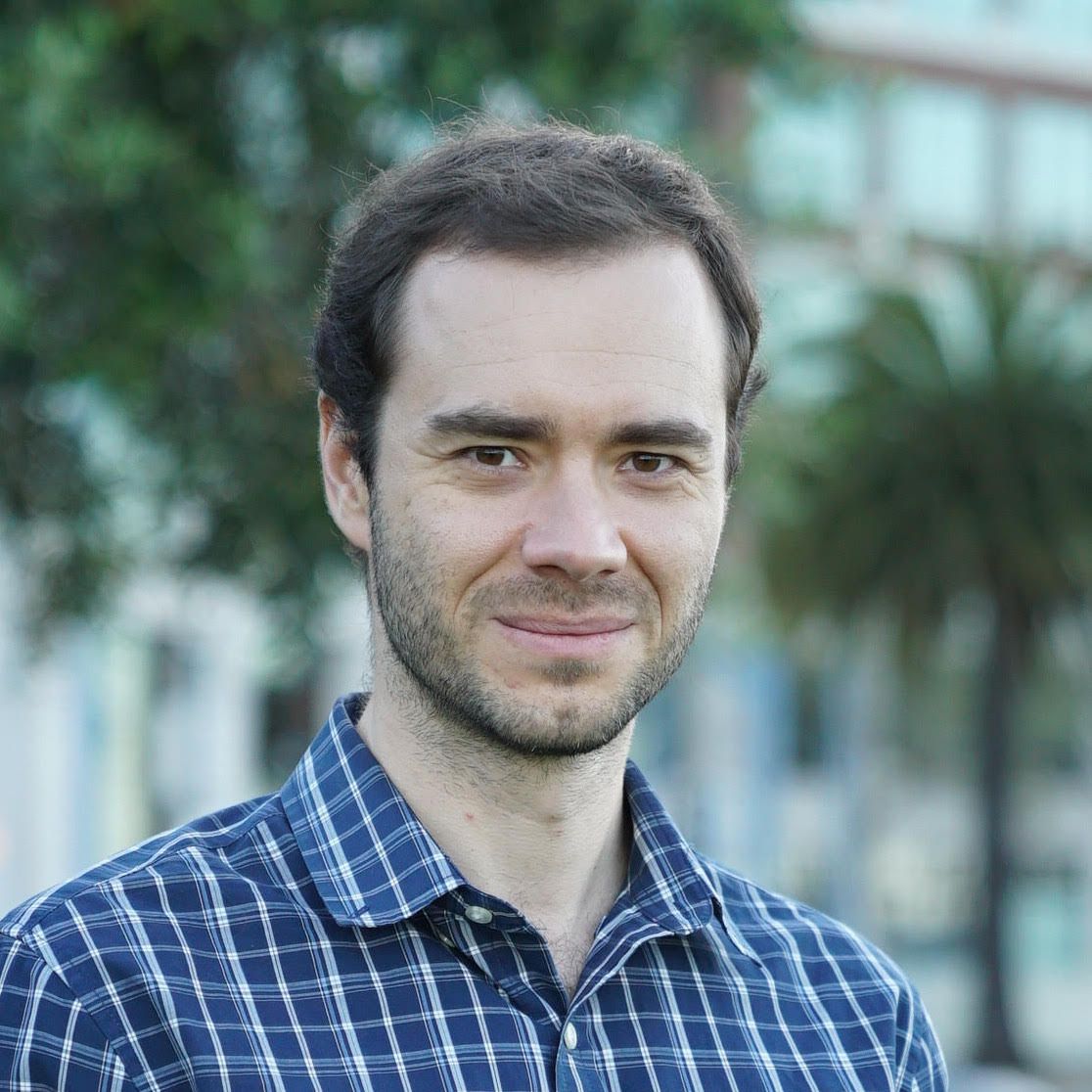
Another indirectly related to “data science” but remarkably comprehensive, original, insightful, and otherwise interesting blog is the writings of Tesla’s AI Director, Andrej Karpathy.
While Andrej primarily covers deep learning, he sometimes ventures into subjects like productivity, education, and even biohacking. (The last one is a fascinating read both in terms of its content and the author’s approach. I know very few people who can take a hobby project and work on it heads down for a year, studying biochemistry, biology, and human nutrition textbooks along the way. Andrej modestly calls it “dipping his toes into biohacking.”)
You can read his blog here. He also has an old medium blog that you can find here.
In addition to those explorations, Andrej has written four extensive “state-of-things” posts about computer vision, software, VR, and ML:
- Software 2.0 (Nov 11, 2017)
- A Peek at Trends in Machine Learning (Apr 7, 2017)
- Virtual Reality: still not quite there, again. (Jan 17, 2017)
- The state of Computer Vision and AI: we are really, really far away. (Oct 22, 2012)
Perhaps the most unique type of content Andrej writes is short fiction stories about AI. Check them out; they’re fascinating:
If you are curious to learn more about his background and how he got into machine learning, read this interview with the with Data Science Weekly on Neural Nets and ConvNetJS from 2014. It’s a bit old, but still very, very good.
My favorite posts from Andrej:
- Doing well in your courses, a guide by Andrej Karpathy
- Deep Neural Nets: 33 years ago and 33 years from now
- Biohacking Lite
P.s. If anyone knows Andrej personally, please urge him to write more!
Peculiar observations
In the process of researching, collecting, and analyzing these blogs in March, I’ve made some peculiar observations that I thought might be of interest. Below is an unordered list of my notes.
- All but one blogs on the list are written by individuals rather than companies. Most company-made content that I’ve found is either of low quality (because most content writers for-hire literally don’t know what they’re talking about, and most founders unfortunately don’t write) and/or is written to satisfy the needs of the company (e.g., more leads! better brand!) rather than the needs of the readers (e.g., insightful, useful information).
- While reading all these blogs, I often had trouble concentrating. Given the abundance of distractions in today’s world and lower attention spans, I assume many people might be experiencing the same issue. What does this mean for content? Will it shift to be more oriented towards passive rather than active consumption (e.g., video)? Does that partly explain TikTok and Twitter?
- Fiction stories is an interesting way to communicate technical knowledge because they bring otherwise abstract knowledge “to the ground.” Examples: one, two, three. Max Tegmark also wrote one as an introduction to his Life 3.0 book.
- Easily skimmable index of all posts is a remarkable way to a) quickly get a sense of the author’s work; and b) find good reading material. More blogs should have them. Some of the indexes I saved:
- https://guzey.com/archive/
- https://erikbern.com/top-posts.html
- https://vickiboykis.com/
- http://www.perceptualedge.com/article-index.php
- https://martinfowler.com/tags/
- http://blog.mikiobraun.de/posts.html
- https://www.evanmiller.org/
- https://madhadron.com/posts_chronological.html
- https://seldo.com/archive
- https://randsinrepose.com/archives/
P.s. You might be wondering why there’s no mention of Narrator Blog in this post. The reason is simple: our blog isn’t yet comprehensive, original, insightful, or otherwise interesting enough to be included. I hope we’ll change that in 2022.